Digital Twins in Biopharma: The Future of Process Validation and Manufacturing Optimization
- Brainz Magazine
- Feb 10, 2020
- 7 min read
Written by: Ashutosh Mahamuni
Digital twin technology is revolutionizing pharmaceutical manufacturing by enabling real-time simulations, predictive modeling, and enhanced process validation. This article explores the role of digital twins in biopharma, their benefits, challenges, and future potential, emphasizing their impact on efficiency, compliance, and cost reduction.
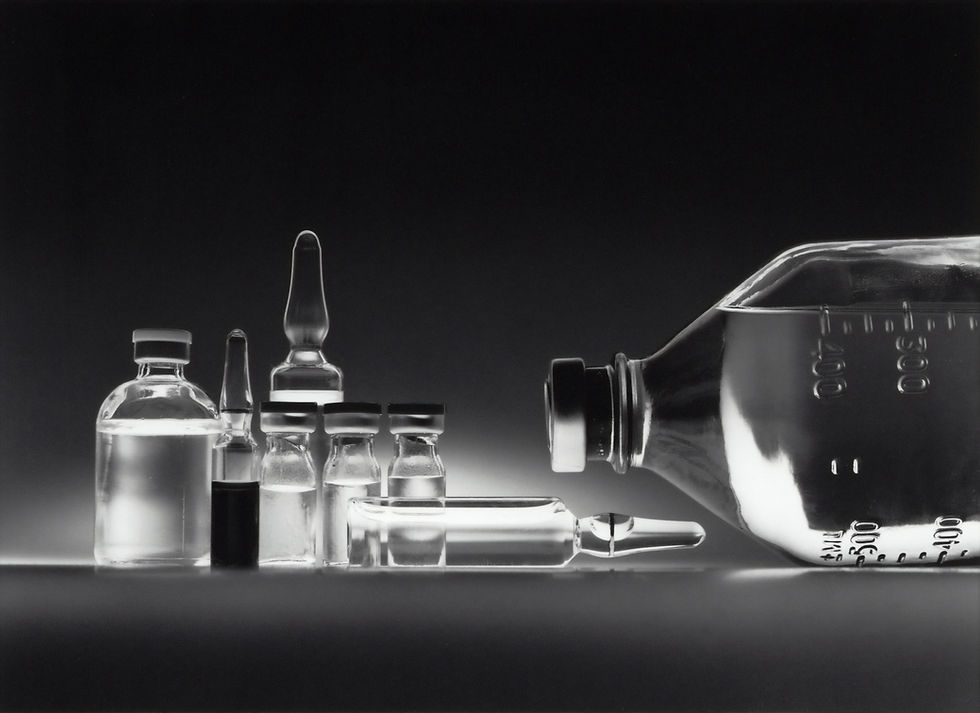
1. Introduction
The pharmaceutical industry is undergoing a digital transformation, and I, Ashutosh Mahamuni, see digital twins as being at the very core of this evolution. From my perspective, digital twins—virtual replicas of physical processes—are revolutionizing how we simulate, monitor, and optimize biopharma production in real time. Their adoption is reshaping process validation, moving it away from traditional trial-and-error methods toward a more data-driven, predictive approach. This shift is not just about efficiency—it's about unlocking a new level of precision and reliability in manufacturing. In this article, I explore the key drivers behind the growing integration of digital twins in the industry, while offering my perspective on their long-term impact.
2. Literature Review: How Digital Twins Work in Biopharmaceutical Manufacturing
Digital twin technology operates by integrating Internet of Things (IoT), artificial intelligence (AI), and machine learning (ML) into biopharma production. These technologies enable:
Real-time data monitoring: Sensors collect data from equipment, providing real-time feedback on performance and deviations.
Simulation and predictive modeling: Digital twins simulate different production scenarios, predicting potential failures before they occur.
Process optimization: Manufacturers can test modifications virtually before implementing them in the physical environment.
Case Studies
Pfizer and Digital Twins: Pfizer has incorporated digital twins to optimize bioreactor conditions, reducing batch failures and improving yield. Implementing digital twin technology in biopharma manufacturing presents multiple challenges, including the need for highly specialized engineers proficient in data analytics, process modeling, and automation. These systems require precise calibration to ensure virtual models accurately replicate real-world bioreactors, necessitating continuous data validation. Additionally, integrating digital twins into existing infrastructure demands significant investment in IoT-enabled sensors, cloud computing, and AI-driven analytics, all of which must comply with GxP (Good Manufacturing Practices) and stringent regulatory standards. Overcoming these hurdles is essential to fully leverage the potential of digital twins in enhancing biopharma production efficiency.
GSK's Implementation: GlaxoSmithKline uses digital twins for continuous monitoring of vaccine production, ensuring quality control and compliance. The implementation of digital twins at this scale requires significant capital investment, including high-performance computing systems, IoT-enabled monitoring devices, and advanced AI-driven analytics platforms. These costs can be substantial, covering specialized hardware, software development, staff training, and regulatory validation. Additionally, the company must hire specialized engineers with expertise in data science, bioprocess modeling, and automation integration to ensure accurate system calibration and real-time responsiveness.
However, despite the high upfront costs, the long-term benefits far outweigh the investment. By reducing batch failures, improving process predictability, and enabling real-time optimizations, GSK is able to significantly cut down production losses and regulatory delays. This not only streamlines vaccine production cycles but also enhances the company's ability to respond quickly to global healthcare needs, ensuring a higher return on investment through operational efficiency and cost savings.
3. Technical Analysis: Key Benefits of Digital Twins in Biopharma
1. Enhanced Process Validation
Predictive analytics improve batch consistency by utilizing real-time data modeling and AI-driven simulations, allowing manufacturers to anticipate and correct process deviations before they occur. Advanced Process Analytical Technology (PAT) tools, such as Raman spectroscopy and near-infrared (NIR) sensors, continuously monitor critical process parameters to ensure uniformity. Companies like Siemens and GE Healthcare have integrated digital twins with predictive modeling software, such as AVEVA Predictive Analytics and Dassault Systèmes BIOVIA, to optimize drug formulation and bioprocessing workflows. However, implementing these systems comes with challenges, including high initial costs, specialized workforce requirements, and regulatory compliance hurdles. Biopharma firms must invest in engineers skilled in computational modeling, AI, and bioprocess automation to fully leverage predictive analytics and digital twins for manufacturing optimization.
2. Real-Time Decision Making which results in cost savings.
In my experience, one of the most powerful aspects of digital twins is their ability to enable real-time decision-making. By continuously monitoring parameters like temperature and pH, these systems can detect early signs of process deviations—often before they escalate into full-scale issues. I’ve seen how teams across engineering, data science, and quality assurance come together to act on these insights, identifying root causes and ensuring all corrective steps meet regulatory standards. This cross-functional responsiveness not only minimizes downtime and waste but also elevates overall product quality.
From a cost perspective, the impact is just as significant. By optimizing formulation and minimizing raw material waste through predictive modeling, digital twins contribute directly to sustainability and bottom-line savings. They also support predictive maintenance, which I find especially valuable. Monitoring equipment health—like tracking agitator vibrations to predict wear—allows for timely servicing, preventing costly breakdowns and production interruptions. Ultimately, digital twins drive smarter manufacturing, where efficiency and quality go hand in hand.
3. Regulatory Compliance
One of the most compelling advantages I’ve seen with digital twins is their ability to enhance traceability and data integrity—something the FDA has been strongly advocating for through its Advanced Manufacturing Technologies (AMT) Program. By integrating digital record-keeping directly into the manufacturing process, digital twins enable secure, real-time tracking of every critical parameter. This not only strengthens compliance with Good Manufacturing Practices (GMP) but also creates a transparent, tamper-proof audit trail that significantly simplifies regulatory inspections and documentation.
In my view, the FDA’s support for digital twins is a clear signal of where the industry is headed. These systems allow manufacturers to simulate and validate processes before actual production, reducing batch failures and ensuring consistency. With AI-driven analytics and automated reporting, digital twins reduce human error and accelerate both internal decision-making and regulatory approvals. It’s a transformative shift—one that brings us closer to a smarter, faster, and more reliable pharmaceutical manufacturing model.
4. Challenges and Considerations
1. Data Security and Privacy:
In my experience, securing sensitive production data is absolutely non-negotiable—especially as digital twins depend on real-time cloud-based infrastructures. The FDA has consistently emphasized the importance of data integrity and compliance with Good Manufacturing Practices (GMP), particularly under 21 CFR Part 11. To meet these expectations, I’ve seen companies invest heavily in robust encryption protocols, detailed audit trails, and tightly controlled access systems. Without these safeguards, the very foundation of digital manufacturing could be compromised.
Cybersecurity risks are very real in biopharma, and the Merck ransomware attack in 2017 is a case I often reference. With losses estimated at $870 million, it was a wake-up call for the entire industry. That’s why I advocate for integrating AI-powered cybersecurity tools and real-time encryption into every layer of a digital twin system. It's not just about protection—it's about ensuring ongoing compliance and resilience in a highly regulated environment.
2. High Implementation Costs
Implementing digital twins is a capital-intensive endeavor, no doubt. From high-performance computing systems and IoT sensors to AI platforms and workforce training, the upfront costs can be steep. But in my view, it's a smart long-term investment. GE Healthcare’s case study is a great example—despite the significant initial expense, they achieved measurable ROI through fewer batch failures, optimized workflows, and reduced downtime. Once systems are in place and aligned with regulatory expectations, the cost savings and efficiency gains speak for themselves.
That said, success depends on seamless integration with existing IT infrastructure. Without that alignment, even the most advanced systems can underperform.
3. Regulatory Adaptation
Regulatory frameworks are evolving, and I see this as a positive sign. The FDA’s Advanced Manufacturing Technologies (AMT) Program is actively shaping guidelines to accommodate innovations like real-time monitoring and AI-based validation. For instance, in continuous bioprocessing, digital twins offer a viable alternative to traditional batch validation. But this shift demands new clarity around data integrity, model transparency, and system validation. I believe clear, adaptive regulation will be key to fully unlocking the potential of digital twins in pharma.
5. Future Trends and Industry Innovations
1. AI-Driven Advancements
From what I’ve seen, the integration of machine learning with digital twins is one of the most promising advancements in biopharma. These algorithms can learn from massive datasets, fine-tuning manufacturing parameters and identifying patterns that would be impossible to catch manually. With AI-assisted digital twins, we’re entering a new era of self-learning systems that adapt to real-time production variations. This capability boosts batch consistency, improves predictive maintenance, and supports automated quality control—all of which significantly reduce downtime and enhance decision-making on the manufacturing floor.
Personally, I believe the self-learning nature of AI-driven twins will become essential as production environments grow more complex and dynamic.
2. Expansion in Personalized Medicine
I’m especially excited about the role digital twins will play in the rise of personalized medicine. Imagine simulating a patient’s biological response to a drug before administering it—that’s where this technology is headed. By integrating genomic and metabolic data, digital twins can help tailor therapies for maximum efficacy and minimal side effects. In oncology, for example, they’re being used to model responses to chemotherapy, potentially leading to more personalized and effective treatment plans.
I also see a major opportunity in cell and gene therapies, where high manufacturing variability demands precise, real-time process control—and digital twins are uniquely positioned to meet that challenge.
But to fully realize this potential, collaboration between regulatory bodies and biopharma companies is essential. From my perspective, the success of digital twins depends on proactive alignment with evolving guidelines. Without it, regulatory uncertainty could slow innovation. I believe that joint efforts will be key in establishing digital twins as a mainstream tool for ensuring quality, speed, and compliance in the future of drug manufacturing.
6. Conclusion
Digital twins are revolutionizing biopharmaceutical process validation and manufacturing optimization. By reducing batch failures, increasing efficiency, and ensuring regulatory compliance, they are setting new standards in the industry. While challenges remain in data security, implementation costs, and regulatory alignment, the future of digital twins in biopharma is promising. From my perspective, the adoption of this technology is not just about improving operational efficiency, but also about reshaping the way pharmaceutical companies approach innovation. Companies that embrace digital twins today will pioneer the next era of pharmaceutical manufacturing, ensuring faster production cycles, enhanced product quality, and greater adaptability to industry challenges.
References
BioProcess International. (2019). The role of digital twins in biopharmaceutical manufacturing. BioProcess International Journal, 17(3), 45-58.
FDA. (2018). Emerging technologies in pharmaceutical manufacturing. U.S. Food and Drug Administration. Retrieved from https://www.fda.gov/biopharma-digital-twins
EMA. (2019). Guidelines for digital validation in pharmaceutical processes. European Medicines Agency. Retrieved from https://www.ema.europa.eu/en/documents/digital-validation
Pfizer. (2019). Case study: Optimizing bioreactor performance with digital twins. Pfizer Manufacturing Reports, 24(2), 89-97.
GlaxoSmithKline. (2018). Continuous monitoring and real-time control in vaccine production. GSK Research Bulletin, 15(4), 33-49.
Author’s Bio
I, Ashutosh Mahamuni, have been steadily building my career in the biopharmaceutical industry with a strong focus on research and advanced manufacturing technologies. My passion lies in biopharmaceutical production and process optimization, where I constantly seek ways to improve efficiency and scalability. While working on Spherofast—an innovative method for continuous and efficient pellet manufacturing—I explored strategies to enhance throughput, minimize material waste, and streamline pharmaceutical workflows. This research naturally led me to a deeper exploration of single-use systems (SUS) in bioprocessing, as their principles closely aligned with the efficiency and sustainability goals I was already pursuing. My engagement with SUS has further expanded my understanding of modern biomanufacturing trends. I am committed to driving innovation in this space, pushing the boundaries of what’s possible in biopharma through smarter, more sustainable production technologies.